-
Full Conference Pass (FC)
-
Full Conference One-Day Pass (1D)
Date: Thursday, December 6th
Time: 9:00am - 10:45am
Venue: Hall D1 (1F, D Block)
Summary: Artists are underrepresented in the reinforcement learning (RL) community due to the steep learning curve involved in in-depth understanding of RL algorithms. However, artists can play an important role in the RL community by defining innovative problems, designing creative environments, and creating novel applications. As a popular tool for artists to experiment with programming, Processing has been highly adapted by many artists as their entry point to programming. Given the popularity of Processing in the creative community, we use this tutorial as a steppingstone to bridge RL and creativity by introducing RL core concepts in Processing. The purpose of this workshop is twofold: 1) to attract more artists to the RL community by demonstrating RL demos in their familiar IDE; 2) to demystify RL problems by implementing them in a high-level language without any external libraries. Importantly, this tutorial is not about introducing a specific programming language, but will focus on how to analyze, frame, and solve RL problems.
Author(s)/Speaker(s):
Moderator: Jieliang Luo, Media Arts & Technology; University of California, Santa Barbara, United States of America
Lecturer(s): Jieliang Luo, Media Arts & Technology; University of California, Santa Barbara, United States of America
Sam Green, Computer Science; University of California, Santa Barbara, United States of America
Author(s)/Speaker(s) Bio:
Jieliang Luo is a researcher and media artists working with reinforcement learning, robotics, and visualization. Currently, he is a Ph.D. candidate and lecturer in Media Arts & Technology at UC Santa Barbara, where he teaches Reinforcement Learning, Data Visualization, and Intelligent Machine Vision. His research focuses on bridging reinforcement learning and creativity by exploring potential simulated and physical platforms for artists, designers, and architects to have easy access to reinforcement learning. He also has worked for Autodesk Robotics Lab as a robotic and machine learning researcher since June, 2018.
Jieliang Luo is a researcher and media artists working with reinforcement learning, robotics, and visualization. Currently, he is a Ph.D. candidate and lecturer in Media Arts & Technology at UC Santa Barbara, where he teaches Reinforcement Learning, Data Visualization, and Intelligent Machine Vision. His research focuses on bridging reinforcement learning and creativity by exploring potential simulated and physical platforms for artists, designers, and architects to have easy access to reinforcement learning. He also has worked for Autodesk Robotics Lab as a robotic and machine learning researcher since June, 2018.
Sam Green is a Ph.D. candidate in Computer Science, at the University of California, Santa Barbara. His research is focused on numerical and architectural optimizations for reinforcement learning, as well as visualization diagnostics for CNN-based RL policies. Prior to attending UCSB, Sam was a Senior Member of Technical Staff, at Sandia National Laboratories, where he gained five years of experience contributing to and leading cryptographic hardware assessment R&D. Sam holds a master's in Applied Math and a bachelor's in Computer Science from the University of Central Arkansas.
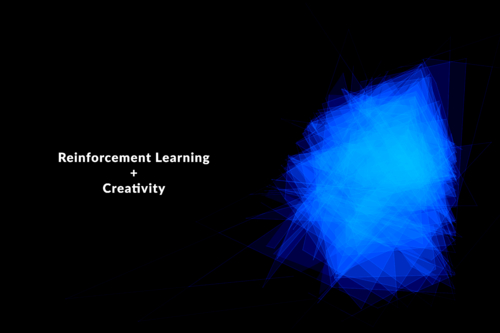