-
Full Conference Pass (FC)
-
Full Conference One-Day Pass (1D)
Date: Wednesday, December 5th
Time: 4:15pm - 6:00pm
Venue: Hall B5(2) (5F, B Block)
Session Chair(s): Hao Li, Pinscreen; University of Southern California, USC Institute for Creative Technologies, United States of America
Warp-Guided GANs for Single-Photo Facial Animation
Abstract: This paper introduces a novel method for realtime portrait animation in a single photo. Our method requires only a single portrait photo and a set of facial landmarks derived from a driving source (e.g., a photo or a video sequence), and generates an animated image with rich facial details. The core of our method is a warp-guided generative model that instantly fuses various fine facial details (e.g., creases and wrinkles), which are necessary to generate a high-fidelity facial expression, onto a pre-warped image. Our method factorizes out the nonlinear geometric transformations exhibited in facial expressions by lightweight 2D warps and leaves the appearance detail synthesis to conditional generative neural networks for high-fidelity facial animation generation. We show such a factorization of geometric transformation and appearance synthesis largely helps the network better learn the high nonlinearity of the facial expression functions and also facilitates the design of the network architecture. Through extensive experiments on various portrait photos from the Internet, we show the significant efficacy of our method compared with prior arts.
Authors/Presenter(s): Jiahao Geng, Zhejiang University, China
Tianjia Shao, Zhejiang University, China
Youyi Zheng, Zhejiang University, China
Yanlin Weng, Zhejiang University, China
Kun Zhou, Zhejiang University, China
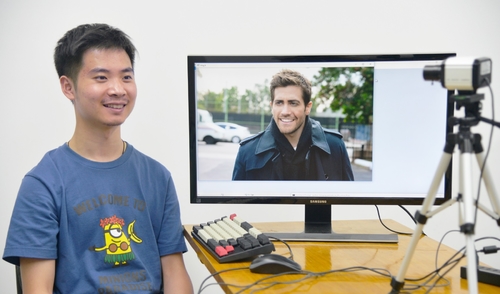
Practical Dynamic Facial Appearance Modeling and Acquisition
Abstract: We present a method to acquire dynamic properties of facial skin appearance, including dynamic diffuse albedo encoding blood flow, dynamic specular BRDF properties as a function of surface deformation, and per frame high resolution normal maps for a sequence of facial performance. The method reconstructs these from a purely passive multi-camera setup, without the need for polarization or requiring to temporally modulate the illumination. It is hence very well suited for integration with existing passive facial performance capture systems. We solve this highly challenging problem by modeling the relationship between skin deformation and surface reflectance, which allows to aggregate information from multiple frames over the course of an actor's performance. We further show that albedo changes due to blood flow are confined to a line in the CIE 1976 Lab color space, and we exploit this subspace for robustly estimating time varying albedo. The presented method is the first system capable of capturing high-quality dynamic appearance maps at full resolution and video framerates, providing a major step forward in the area of facial appearance acquisition.
Authors/Presenter(s): Paulo Gotardo, Disney Research, Switzerland
Jeremy Riviere, Disney Research, Switzerland
Derek Bradley, Disney Research, Switzerland
Abhijeet Ghosh, Imperial College London, United Kingdom
Thabo Beeler, Disney Research, Switzerland
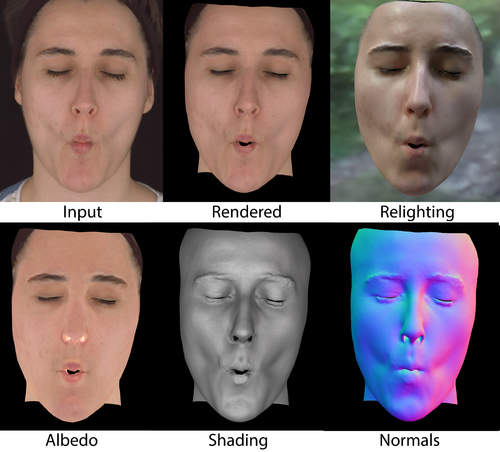
Stabilized Real-time Face Tracking via a Learned Dynamic Rigidity Prior
Abstract: Despite the popularity of real-time monocular face tracking systems in many successful applications, one overlooked problem with these systems is rigid instability. It occurs when the input facial motion can be explained by either head pose change or facial expression change, creating ambiguities that often lead to jittery and unstable rigid head poses under large expressions. Existing rigid stabilization methods either employ a heavy anatomically-motivated approach that are unsuitable for real-time applications, or utilize heuristic-based rules that can be problematic under certain expressions. We propose the first rigid stabilization method for real-time monocular face tracking using a dynamic rigidity prior learned from realistic datasets. The prior is defined on a region-based face model and provides dynamic region-based adaptivity for rigid pose optimization during real-time performance. We introduce an effective offline training scheme to learn the dynamic rigidity prior by optimizing the convergence of the rigid pose optimization to the ground-truth poses in the training data. Our real-time face tracking system is an optimization framework that alternates between rigid pose optimization and expression optimization. To ensure tracking accuracy, we combine both robust, drift-free facial landmarks and dense optical flow into the optimization objectives. We evaluate our system extensively against state-of-the-art monocular face tracking systems and achieve significant improvement in tracking accuracy on the high-quality face tracking benchmark. Our system can improve facial-performance-based applications such as facial animation retargeting and virtual face makeup with accurate expression and stable pose. We further validate the dynamic rigidity prior by comparing it against other variants on the tracking accuracy.
Authors/Presenter(s): Chen Cao, Snap Inc., United States of America
Menglei Chai, Snap Inc., United States of America
Oliver Woodford, Snap Inc., United States of America
Linjie Luo, Snap Inc., United States of America
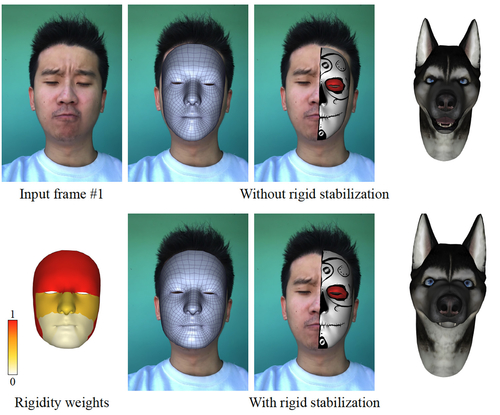
Deep Incremental Learning for Efficient High-Fidelity Face Tracking
Abstract: In this paper, we present an incremental learning framework for efficient and accurate facial performance tracking. Our approach is to alternate the modeling step, which takes tracked meshes and texture maps to train our deep learning-based statistical model, and the tracking step, which takes predictions of geometry and texture our model infers from measured images and optimize the predicted geometry by minimizing image and geometric errors. Our Geo-Tex VAE model extends the convolutional variational autoencoder for face tracking, and jointly learns and represents deformations and variations in geometry and texture from tracked meshes and texture maps. To accurately model variations in facial geometry and texture, we introduce the decomposition layer in the Geo-Tex VAE architecture which decomposes the facial deformation into global and local components. We train the global deformation with a fully-connected network and the local deformations with convolutional layers. Despite running this model on each frame independently -- thereby enabling a high amount of parallelization -- we validate that our framework achieves sub-millimeter accuracy on synthetic data and outperforms existing methods. We also qualitatively demonstrate high-fidelity, long-duration facial performance tracking on several actors.
Authors/Presenter(s): Chenglei Wu, Facebook Reality Labs, United States of America
Takaaki Shiratori, Facebook Reality Labs, United States of America
Yaser Sheikh, Facebook Reality Labs, United States of America
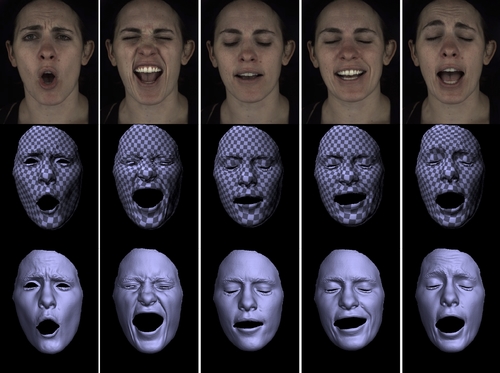